Soil Hydrology Model (SHM)
Regional-scale atmospheric models require the initialization of SW (often
referred to as soil moisture) at various layers in the soil over the horizontal
model domain. Routine SW measurements over large areas (like the ones typically
covered by regional-scale model domains) are unfeasible. Therefore, climatological
estimates of SW have often been used to provide initial conditions to the
regional models. Unfortunately, this approach may result in large errors
during periods of excessive rainfall or drought. In the absence of observations,
an indirect method is needed to determine SW fields from routine meteorological
observations. We have taken two approaches to this: 1) remote sensing (described
previously), which we refer to as "top-down", and 2) use of a
soil hydrology model (SHM), which we refer to as "bottom up".
The original objective of our study was to combine the two approaches, specifically
by using the remote measurements to "nudge" the SHM. We now discuss
the bottom-up method and the limitations in the nudging approach.
The SHM, developed by Capehart and Carlson (1994), uses a one-dimensional
SW diffusion and gravitation scheme to determine the temporal evolution
of SW as a function of depth. It is driven entirely by routine meteorological
observations at the surface (i.e., the atmospheric forcing: air temperature,
moisture and wind speed, as well as cloud cover and precipitation), information
on soil and land cover characteristics (soil texture, land cover type, and
fractional vegetation cover), and terrain slope. It includes modules for
precipitation, interception of rainwater by the vegetation, ponding, infiltration,
surface and subsurface runoff, subsurface diffusion, ET, and some rudimentary
vegetation mechanics. The soil column depth, the vertical grid spacing within
the soil, and the nature of the lower boundary (impermeable or saturated
barrier) in the model are specified by the user.
The SHM is initialized with an arbitrary SW profile, usually chosen to be
50% of saturation. The model, driven by the atmospheric forcing, converges
toward a common solution regardless of the initial SW value. This convergence
takes place over a period ranging from a few weeks to a couple of months,
depending on the precipitation amounts. We call this period of adjustment
the "balancing" period.
Linkage of the SHM with the "Top-Down" Approach
Use of remote sensing to nudge the SW estimates provided by the SHM has
encountered a theoretical block. The "triangle method" (Figure
13) implies the prevalence of very dry soil patches even during periods
of relatively abundant rainfall. Conversely, there are also many pixels
with high SW even in arid regions. Moreover, validation studies (Perry and
Carlson 1988; Gillies et al., 1995b) indicate that the remotely derived
SW values are always lower than those measured by in-situ methods or by
microwave techniques, even when the correlation between the two data sets
is high. Preliminary analyses by Humes and Kustas at USDA indicate that
this is a real effect. The lack of correlation between thermal and microwave
estimates of SW is, at first glance, perturbing but it does suggest some
interesting insights into the manner in which soil dries. Our current modeling work suggests that soil crusting plays a role in the surface drying.
When a surface dries out, the hydraulic conductivity decreases rapidly,
thereby forming a seal for the water below. This allows the surface to become
relatively warm despite relatively elevated SW at deeper layers.
Crusting occurs selectively when there is a range of soil properties. Figure
15 shows that even within a single soil class (silt loam) the normal variance
of soil properties will result in parts of the surface drying out very rapidly
following a rainstorm, while other areas remain wet for days. The significance
of this finding is that surface radiant temperature measurements (
)
are incapable of providing reliable information on the root zone SW.
This is the reason why surface radiant temperatures may not be useful for
subsurface hydrology, although it is clear that the surface moisture content
is closely tied to the surface energy balance. Indeed, it is clear that
Fr and Mo are the two dominant variables governing the surface energy balance.
As such they are also important parameters for inclusion in the SHM and
the MM, although the moisture availability would nevertheless pertain only
to a surface layer.
Linkage of the SHM with the Mesoscale Model
In order to use the SHM to provide SW initial conditions for the land-surface
component of the MM (i.e., BATS), the SHM must be applied to each grid cell
over the entire MM domain. Smith et al. (1994) developed and tested this
procedure (called the SHM system) which is illustrated in Figure 16. This
system comprises three major components: the preprocessing programs, MIXER,
and DRIVER. The preprocessing programs (some of which are part of the MM
modeling system) use topographic information to provide gridded terrain
heights for the domain of interest, and perform an objective analysis of
surface meteorological data over the model grid, which results in the atmospheric
forcing dataset. MIXER incorporates the atmospheric forcing dataset, the
gridded soil- and land cover-characteristics datasets and the terrain-slope
dataset, calculates the necessary radiation and ET variables required by
the SHM, and produces the complete gridded dataset that is used by DRIVER.
DRIVER then runs the SHM for each grid cell and produces the SW fields required
by the MM. The SHM was modified so that it uses the same soil and land cover
characteristics as BATS.
Smith et al. (1994) tested the SHM-MM linkage, where the SHM system
was driven by a four-and-a-half-month historical meteorological dataset
for three nested MM domains centered on the Mahantango Creek Watershed (MCW)
in the SRB (Figure 17). Each domain contains a 60 by 60 array of grid cells
with cell dimensions of 36-km, 12-km, and 4-km, respectively for the three
domains. The MM was then initialized with the SHM-produced SW fields and
a 12-hour simulation was made with the MM. A five-minute video has been
prepared which demonstrates the time evolution of the SW fields over the
36-km SRBEX domain as generated by the SHM for that same period. This tape
is available for demonstration (copy available on loan).
We are currently developing a real-time version of the SHM system, which
will soon provide up-to-date SW fields to MM5 (Lakhtakia et al., 1994a,b).
This will allow for real-time testing of the SHM-system capabilities when
linked to MM5. Testing will include MM5 simulations, using the SHM-simulated
SW fields as initial conditions, and comparison of the predicted afternoon
temperature and specific humidity close to the surface with observations.
We will also use the method of Mahfouf (1991) in which errors in the forecast
variables are used to correct the SW used as MM5 initial conditions.
We have also begun (in collaboration with Dr. Jimy Dudhia of NCAR) to gather
the information to create a multilayer SW climatology using the SHM on a
rectangular 18-km grid covering the 48 conterminous US, for the period 1979
through 1995. Available in-situ measurements of SW will be used to assess
the accuracy of the SHM output.
Land Cover and Soils Information
As discussed previously, surface characteristics are an important component
of the modeling effort within SRBEX. The SHM and the MM both require the
specification of several landcover parameters for each grid cell within
the domain. This is achieved by specifying one of 18 land cover types, which,
with the help of a lookup table, provide the land cover characteristics
re quired by both models (e.g., roughness length, depth of rooting-zone
soil layer, vegetation albedo, minimum stomatal resistance, etc.).
Until recently, the only high-resolution land cover dataset routinely used
in the MM was the one archived at NCAR, which covers the entire globe at
10 arc-minute resolution (approximately 19-km). In order to initialize the
SHM and the BATS module within the MM, this dataset (hereafter referred
to as the NCAR dataset) has to be converted to the appropriate BATS land
cover type. Figure 18 shows the land cover type distribution over the 4-km
SRBEX domain using the NCAR dataset.
The Land Cover Characteristics Database developed at the United States Geological
Survey EROS Data Center (Loveland et al., 1991) (hereafter referred to as
the EDC database), has also been used in preliminary SRBEX studies. This
database provides land cover types for the entire 48 conterminous United
States at 1-km resolution. The original 167 land cover classes in the EDC
database were reduced to the 18 BATS land cover types, and analyzed to the
MM horizontal nested domains using a modal aggregation technique. Land cover
information for the areas of Canada that are part of the 36-km and the 12-km
MM domains was merged from the NCAR dataset. This resulted in seamless land
cover maps for each of the domains. Figure 18 also shows the land cover
type distribution over the 4-km domain using the EDC database. The modeling
results using the EDC database were presented at the USGS-organized workshop:
"Test and Evaluation of the USGS 1-km AVHRR Land-Cover Characteristics
Data for the Conterminous United States: Results and Recommendations",
that took place in April 1994 at the EROS Data Center, in Sioux Falls, South
Dakota.
Apart from information on the land cover type, the SHM and the MM also require
the specification of the soil texture (one of the 12 USDA soil texture classes)
for each grid cell in the MM domain. The soil texture, with the help of
a lookup table, provides the soil parameters required by both models (e.g.,
SW at saturation, minimum soil suction, saturated hydraulic conductivity,
SW at the wilting point, etc.). Traditionally, the lack of reliable information
on soil characteristics at the regional scale has been an impediment to
SVAT improvement. In fact, until now the SHM and the BATS module within
the MM have relied on soil texture derived from land cover. Figure 19 shows
the soil texture distribution over the 4-km domain derived from the NCAR
land cover and the EDC land cover.
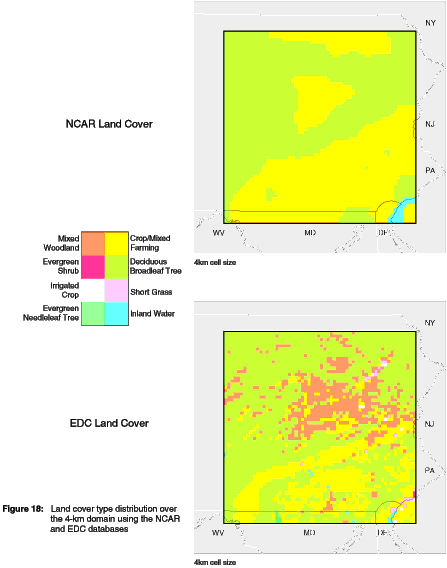
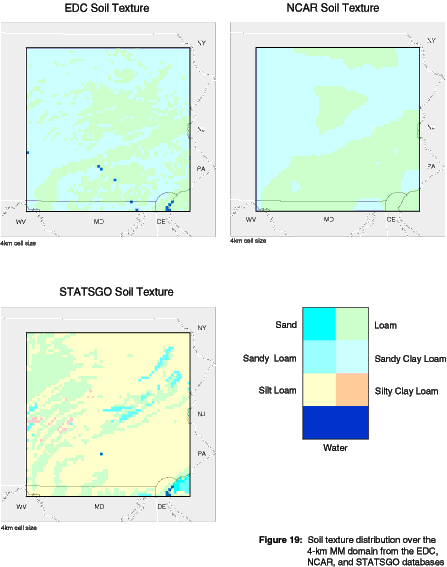
The recent development of the State Soil Geographic Database (STATSGO)
by the USDA Natural Resource Conservation Service (NRCS) shows potential
for the delivery of much needed realistic soil information to the modeling
community (Miller and Lakhtakia, 1994a, b). STATSGO has been developed for
river basin, multi-state, state, and multi-county resource planning. The
compiled soil maps are created with the USGS 1:250,000-scale topographic
quadrangles as base maps. STATSGO contains information on a wide range of
soil properties (e.g., texture, particle size distribution, available water
capacity, and bulk density). Initial work with STATSGO has produced a soil
texture dataset that is compatible with the lookup table approach of the
models. Figure 19 shows the STATSGO-derived soil texture distribution over
the 4-km MM domain.
Two simulations with the SHM, using the atmospheric forcing dataset from
Smith et al. (1994), were performed to test the effect of soil texture information
on the SHM results. While in both simulations the SHM used the EDC land
cover information, for the first simulation it used the EDC soil texture
information and for the second the STATSGO soil texture information. The
last ten days of the period over which the SHM simulations were performed
(9 July - 18 July 1990) coincide with the NASA Multisensor Airborne Campaign
(MAC-HYDRO '90), which was coordinated by Dr. E. T. Engman of NASA Goddard
Space Flight Center. This campaign took place over the Mahantango Creek
Watershed, which is located in the geographical center of the SRBEX model
domains. The campaign area contained 27 sites where SWC measurements were
taken with neutron-probe and electromagnetic soil-moisture measuring devices.
Instrument readings of SWC were integrated over 15-cm and 30-cm depths from
the surface. Most of the probe sites were in a meadow environment, with
some probes placed in corn fields as well. The SHM output from both simulations
from the 4-km domain central grid cell are compared with the averaged field
measurements for the upper 15-cm soil layer (Figure 20). The overall pattern
of the SHM results from both simulations is consistent with the field measurements. However, the results from the second simulation show much better
agreement with the observations (Figure 21).
Table of Contents
Previous Section
Next Section
Download Report
Graphics and HTML text markup by Phil Kolb
Comments to: R. White, raw@essc.psu.edu